Unlocking Insights With The Generalized Method Of Moments
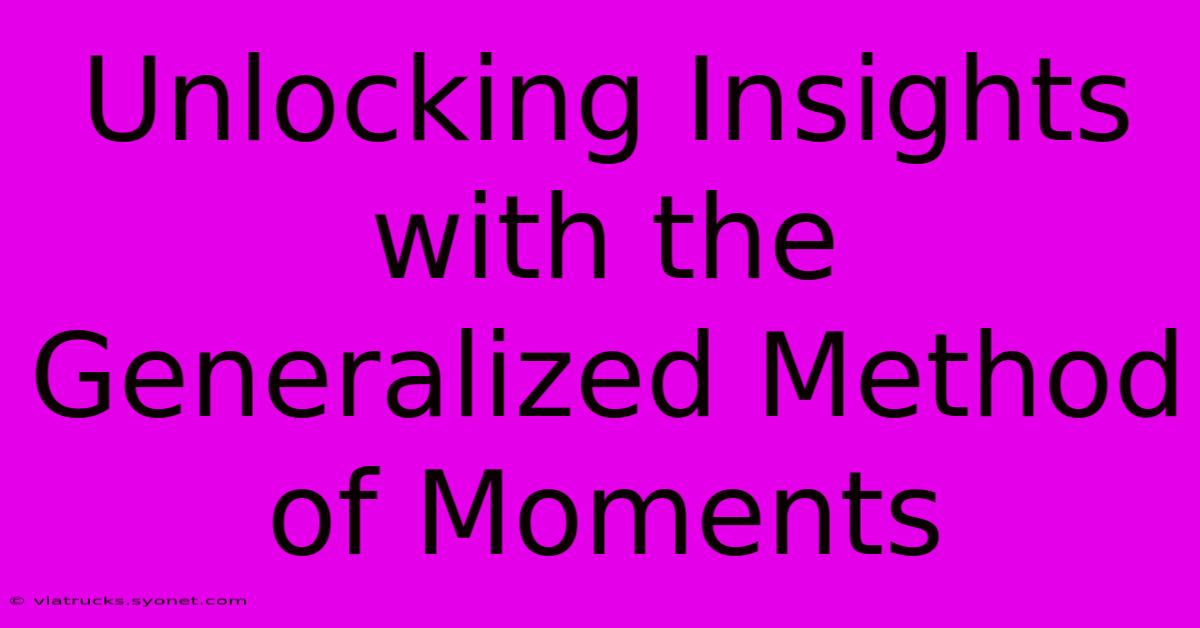
Table of Contents
Unlocking Insights with the Generalized Method of Moments (GMM)
The Generalized Method of Moments (GMM) is a powerful statistical technique used to estimate parameters in econometric models and other fields where data might be complex or incomplete. Unlike traditional methods like Ordinary Least Squares (OLS), GMM doesn't require a fully specified likelihood function, making it incredibly versatile and applicable to a wide range of situations. This article will explore the core concepts of GMM, its advantages, and its practical applications.
Understanding the Fundamentals of GMM
At its heart, GMM leverages the idea of moment conditions. These are theoretical relationships between the model's parameters and the data's moments (e.g., mean, variance, etc.). The method aims to find parameter estimates that best satisfy these moment conditions, even in the presence of misspecification or unknown distributions.
Here's a breakdown of the key steps involved:
-
Moment Conditions: Define a set of population moment conditions, which are equations that should hold true if the model's parameters are correctly specified. These conditions typically involve expectations of functions of the data and the parameters.
-
Sample Moments: Calculate the sample equivalents of these moment conditions using your observed data. These will naturally differ from the population moments due to sampling error.
-
Estimation: GMM aims to find parameter estimates that minimize a measure of the discrepancy between the sample moments and the theoretical moment conditions. This is often achieved using a weighted distance metric. The optimal weighting matrix is crucial for efficient estimation and is usually estimated iteratively.
-
Inference: Once the parameter estimates are obtained, GMM provides methods for assessing their statistical significance and constructing confidence intervals. This often involves using asymptotic theory.
Advantages of Using GMM
GMM offers several significant advantages over other estimation methods:
-
Robustness: GMM is robust to misspecification of the error distribution. This is a major benefit compared to maximum likelihood estimation (MLE), which requires a correct specification of the likelihood function.
-
Flexibility: It can handle models with over-identifying restrictions, where there are more moment conditions than parameters. This allows for testing the validity of the model's assumptions.
-
Efficiency: Under correct specification, GMM estimators can be asymptotically efficient. The choice of the weighting matrix plays a crucial role in achieving this efficiency.
-
Wide Applicability: GMM finds applications in various fields, including economics, finance, and biostatistics, for estimating dynamic panel data models, models with endogenous variables, and models with weak instruments.
GMM in Practice: Applications and Examples
GMM is frequently used in several contexts:
-
Dynamic Panel Data Models: GMM is a popular choice for estimating dynamic panel data models, where the dependent variable is influenced by its own past values. This is common in economic analyses of investment, consumption, and growth.
-
Models with Endogenous Variables: When independent variables are correlated with the error term (endogeneity), OLS estimators are biased and inconsistent. GMM offers a way to consistently estimate parameters in such situations.
-
Instrumental Variables (IV) Estimation: GMM generalizes instrumental variable estimation, accommodating situations where there are more instruments than endogenous variables.
-
Generalized Empirical Likelihood (GEL): GEL is a class of estimators closely related to GMM, offering alternative ways to minimize the distance between sample and population moments.
Limitations and Considerations
While GMM is a powerful tool, it also has limitations:
-
Computational Complexity: The iterative nature of GMM estimation can be computationally intensive, particularly with large datasets or complex models.
-
Sensitivity to Weighting Matrix: The choice of the weighting matrix significantly impacts the efficiency and properties of the GMM estimator. An incorrectly specified weighting matrix can lead to inefficient or inconsistent estimates.
-
Assumption of Correctly Specified Moment Conditions: The validity of GMM relies heavily on the correctness of the specified moment conditions. Misspecified moment conditions can lead to biased and misleading results.
Conclusion
The Generalized Method of Moments represents a powerful and flexible technique for parameter estimation in a wide variety of statistical models. Its robustness to distributional assumptions and ability to handle endogeneity make it a valuable tool for researchers across many disciplines. However, careful consideration of its assumptions, computational aspects, and the choice of the weighting matrix is crucial for accurate and reliable results. Understanding these nuances allows researchers to unlock the valuable insights offered by this versatile method.
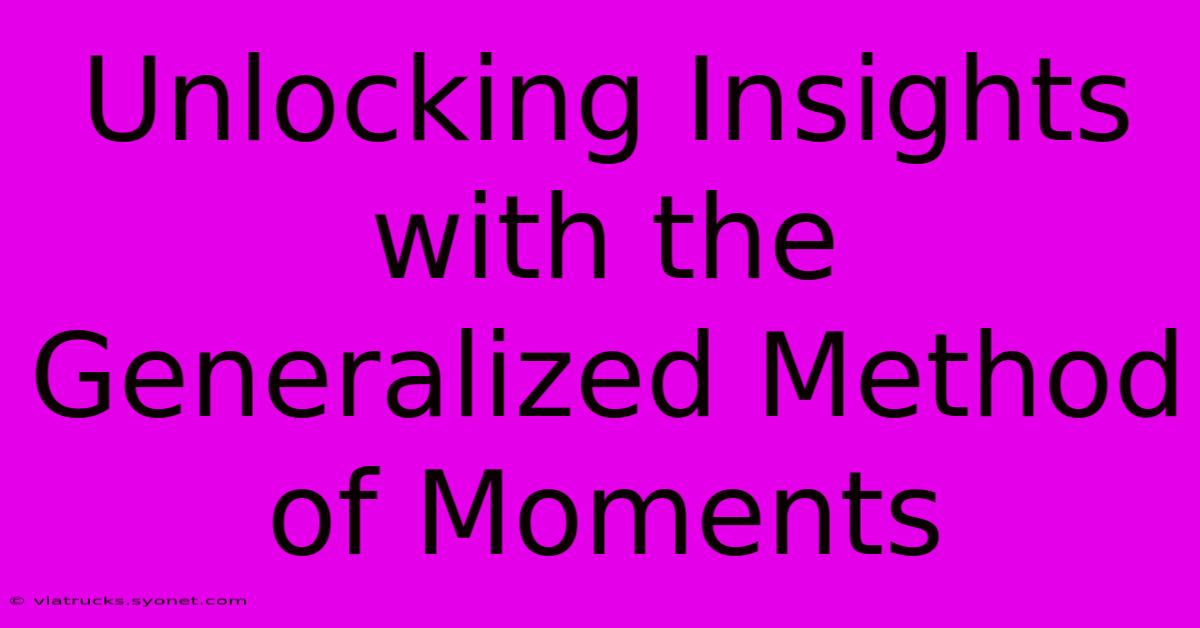
Thank you for visiting our website wich cover about Unlocking Insights With The Generalized Method Of Moments. We hope the information provided has been useful to you. Feel free to contact us if you have any questions or need further assistance. See you next time and dont miss to bookmark.
Featured Posts
-
Tired Of The Hustle St Johns Wood Offers A Peaceful Retreat
Feb 09, 2025
-
Beyond The Hype Chiefs Vs Bills Player Stats Analysis
Feb 09, 2025
-
Beyond Baseball Exploring The Tohoku Rakuten Golden Eagles Phenomenon
Feb 09, 2025
-
The Ultimate Guide To The Fairy Tail 100 Year Quest Manga
Feb 09, 2025
-
Your Dream Island Getaway Explore Prince Of Wales Island
Feb 09, 2025