The Secret Weapon For Robust Statistical Analysis: GMM
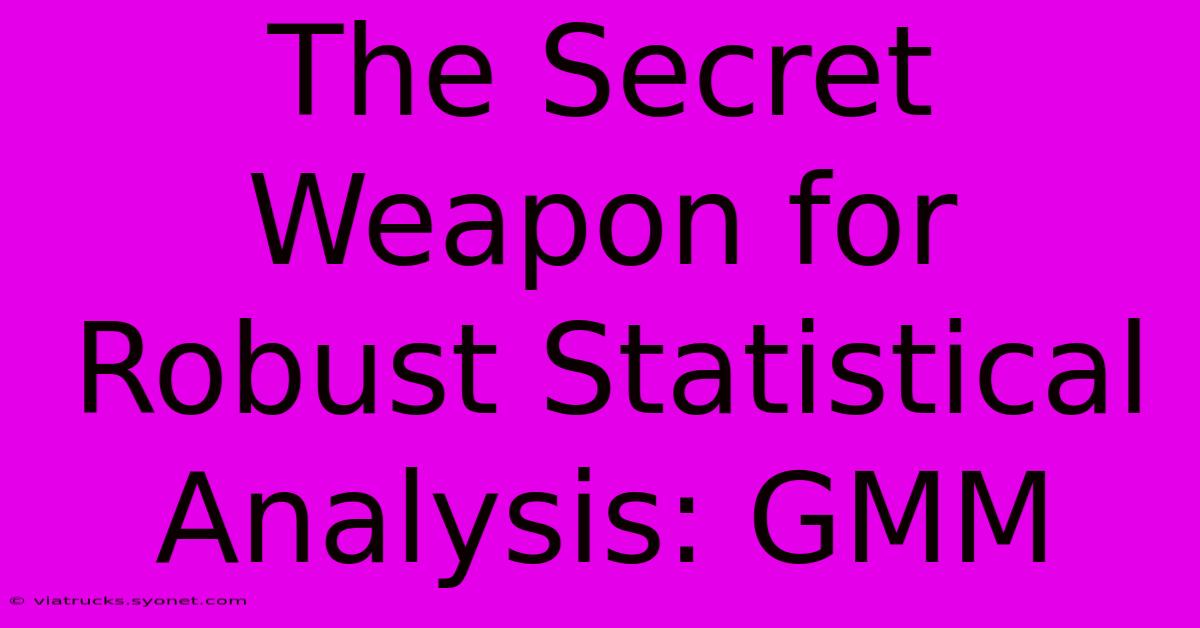
Table of Contents
The Secret Weapon for Robust Statistical Analysis: Generalized Method of Moments (GMM)
Generalized Method of Moments (GMM) is a powerful and versatile statistical technique used to estimate parameters in econometrics, finance, and other fields. While not as widely known as some other methods like Ordinary Least Squares (OLS), GMM offers a significant advantage: robustness. This article delves into the core principles of GMM, highlighting its strengths and showcasing its utility in situations where traditional methods fall short.
Understanding the Power of GMM
GMM is particularly valuable when dealing with complex models and weak assumptions about the data generating process. Unlike OLS, which relies on strong assumptions like homoscedasticity and no autocorrelation, GMM only requires that the model's moment conditions are correctly specified. These moment conditions are essentially population expectations that should hold true if the model is correctly specified.
What are Moment Conditions?
Moment conditions are equations that describe the relationships between the model's parameters and the data. They are derived from the underlying economic theory or assumptions about the data-generating process. For example, in a model of asset pricing, a moment condition might state that the expected value of the residuals (the difference between actual returns and predicted returns) should be zero.
The GMM Estimation Process
GMM works by finding parameter estimates that minimize a distance metric between the sample moments and their theoretical counterparts (the moment conditions). This is achieved through an iterative process that involves:
- Choosing moment conditions: This step is crucial and requires careful consideration of the model's structure and the available data.
- Calculating sample moments: This involves computing sample averages of the functions defined by the moment conditions.
- Minimizing the distance: GMM employs a weighting matrix to weigh the deviations between sample and theoretical moments, and finds the parameter estimates that minimize this weighted distance. This weighting matrix is crucial for efficiency; optimal weighting often involves an iterative process.
- Testing the model: GMM provides diagnostic tests, like the J-test, to assess the validity of the over-identifying restrictions. These tests help determine if the chosen moment conditions are consistent with the data.
Advantages of GMM over Other Methods
- Robustness to misspecification: GMM is less sensitive to violations of assumptions about the error terms than OLS.
- Handles endogeneity: GMM can handle endogenous variables (variables correlated with the error term), a common problem in econometric modeling. Instrumental variables are often used in this context.
- Flexibility: GMM can be applied to a wide range of models, including nonlinear models and models with limited dependent variables.
Applications of GMM
GMM is widely used in various fields:
- Econometrics: Estimating dynamic panel data models, analyzing simultaneous equations, and handling endogeneity.
- Finance: Pricing assets, estimating risk models, and analyzing portfolio performance.
- Microeconomics: Estimating production functions, analyzing consumer behavior, and studying market structures.
Limitations of GMM
While powerful, GMM has limitations:
- Computational intensity: The iterative estimation process can be computationally demanding, especially with large datasets or complex models.
- Sensitivity to weighting matrix: The efficiency of GMM estimates depends heavily on the choice of the weighting matrix.
- Potential for weak instruments: When using instrumental variables, weak instruments can lead to imprecise and unreliable estimates.
Conclusion: Unlocking the Power of Robust Analysis
GMM represents a significant advancement in statistical analysis, offering robustness and flexibility that traditional methods lack. By understanding its principles and limitations, researchers can leverage its power to tackle complex problems and gain more reliable insights from their data. While requiring a deeper understanding of statistical theory than simpler methods, the potential rewards – in the form of robust and reliable estimates – make mastering GMM a worthwhile endeavor for anyone conducting serious quantitative analysis. Its applicability across diverse fields underlines its status as a truly indispensable tool in the statistician's arsenal.
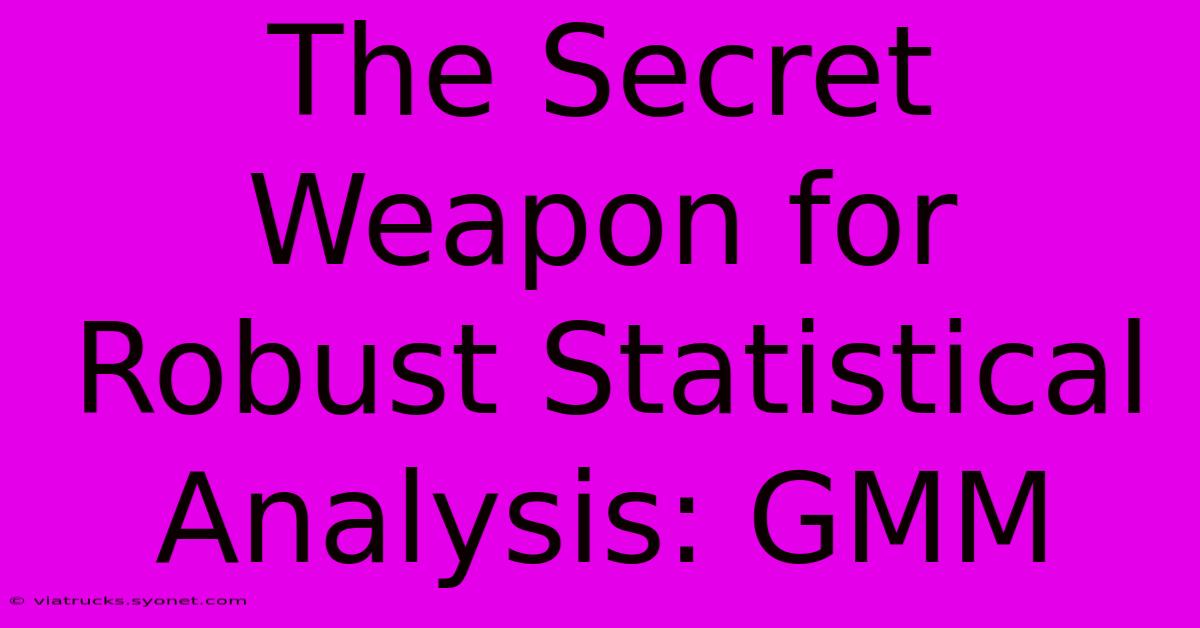
Thank you for visiting our website wich cover about The Secret Weapon For Robust Statistical Analysis: GMM. We hope the information provided has been useful to you. Feel free to contact us if you have any questions or need further assistance. See you next time and dont miss to bookmark.
Featured Posts
-
Stress Less How God Grant Me The Serenity Can Change Your Life
Feb 10, 2025
-
Analyzing Liverpools Fa Cup Loss
Feb 10, 2025
-
Jar Of Flies The Story Behind Aics Masterpiece
Feb 10, 2025
-
Chickamauga And Chattanooga A Must See Civil War Battlefield
Feb 10, 2025
-
Solve The Mystery Decoding The Jaguars Eagles Match With Stats
Feb 10, 2025