Solve The Estimation Puzzle With The Generalized Method Of Moments
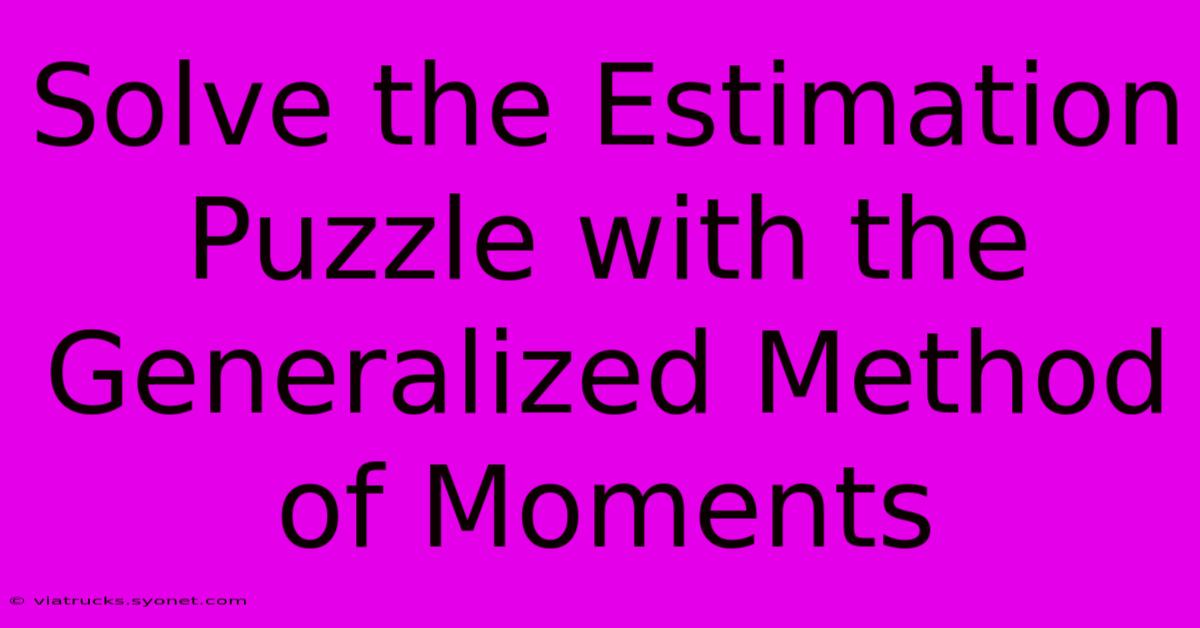
Table of Contents
Solve the Estimation Puzzle with the Generalized Method of Moments (GMM)
The Generalized Method of Moments (GMM) is a powerful statistical technique used to estimate parameters in econometrics and other fields where you're dealing with complex models and potentially noisy data. It's particularly useful when you have more moment conditions than parameters, providing a robust way to handle estimation challenges that simpler methods like Ordinary Least Squares (OLS) can't easily address. This article will delve into GMM, explaining its workings, advantages, and limitations.
Understanding the Core of GMM
At its heart, GMM leverages the concept of moment conditions. These are statements about the expected value of certain functions of your data and the unknown parameters. For instance, if you're modeling consumption, a moment condition might state that the expected value of the difference between actual consumption and its predicted value (based on your model) should be zero.
The beauty of GMM is its ability to use multiple moment conditions simultaneously. This is crucial when dealing with models where standard assumptions, like those needed for OLS, are violated. The method cleverly finds the parameter estimates that best satisfy all these moment conditions, minimizing a weighted distance between the sample moments and their theoretical counterparts.
The GMM Estimation Process: A Step-by-Step Guide
-
Specify the Model: Define the model you're trying to estimate, including the parameters you want to find.
-
Formulate Moment Conditions: Identify a set of moment conditions that should hold if your model is correctly specified. These conditions are typically based on theoretical economic principles or assumptions about the data generating process.
-
Calculate Sample Moments: Compute the sample analogs of the theoretical moment conditions using your observed data.
-
Choose a Weighting Matrix: This matrix determines the relative importance given to different moment conditions. An optimal weighting matrix leads to efficient parameter estimates. The initial weighting matrix is often an identity matrix, followed by iterative re-weighting.
-
Minimize the GMM Objective Function: GMM finds the parameter estimates that minimize a quadratic form involving the sample moments, their theoretical counterparts, and the weighting matrix. This minimization process usually involves numerical optimization techniques.
-
Iterate (if necessary): With the optimal weighting matrix, the process often iterates between steps 4 and 5 to improve the efficiency of the estimates.
-
Obtain Parameter Estimates and Standard Errors: The optimized parameters provide the GMM estimates, while the estimated covariance matrix provides their standard errors, crucial for hypothesis testing.
Advantages of Using GMM
-
Robustness: GMM is robust to heteroskedasticity and autocorrelation, common problems in econometric data. It doesn't require stringent assumptions about the error terms.
-
Handles Overidentification: When you have more moment conditions than parameters (overidentification), GMM provides a way to test the validity of your model. The J-test assesses whether the overidentifying restrictions are consistent with the data. A significant J-statistic suggests misspecification of the model.
-
Flexibility: GMM can handle various model specifications and data structures.
Limitations of GMM
-
Computational Intensity: GMM estimation often requires iterative numerical optimization, which can be computationally intensive, particularly with large datasets or complex models.
-
Sensitivity to Weighting Matrix: The choice of weighting matrix can affect the efficiency of the estimates. An inappropriate choice can lead to inefficient or even inconsistent estimates.
-
Weak Instruments: If the moment conditions are weakly correlated with the parameters, GMM estimators can exhibit poor finite sample properties, leading to inaccurate estimates and high standard errors.
Conclusion: GMM – A Powerful Tool for Estimation
The Generalized Method of Moments offers a powerful and versatile approach to parameter estimation in situations where traditional methods are inadequate. Its ability to handle multiple moment conditions, robustness to misspecification, and capacity for model testing make it a valuable tool in many fields, particularly econometrics. However, understanding its computational requirements and potential limitations is crucial for its effective and appropriate application. Careful consideration of the weighting matrix and assessment of the J-test are vital for obtaining reliable and meaningful results.
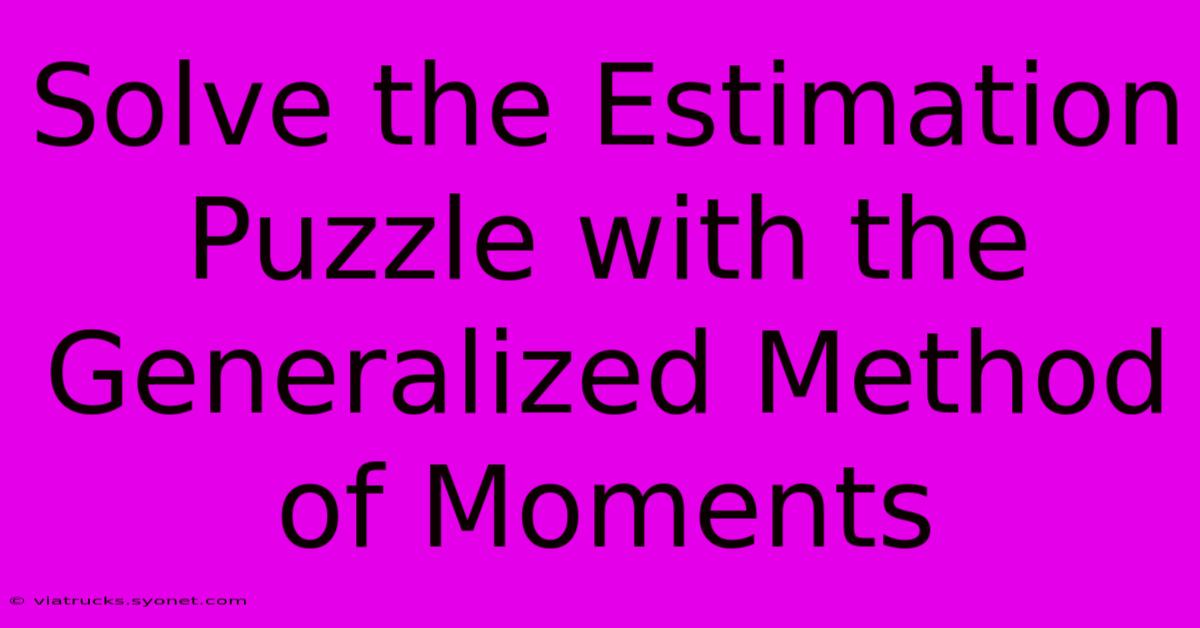
Thank you for visiting our website wich cover about Solve The Estimation Puzzle With The Generalized Method Of Moments. We hope the information provided has been useful to you. Feel free to contact us if you have any questions or need further assistance. See you next time and dont miss to bookmark.
Featured Posts
-
Uncover The Secrets I Know What You Did Last Summer Book
Feb 09, 2025
-
Just The 10 Of Us The Perfect Size For An Unforgettable Celebration
Feb 09, 2025
-
Confused About Grand Marnier Mystery Solved
Feb 09, 2025
-
Ravens Steelers Surprise Standouts Revealed In Player Stats Breakdown
Feb 09, 2025
-
Fa Cup Chelsea Loses To Brighton
Feb 09, 2025