Pointwise Mutual Information: The Secret To Deeper Data Analysis
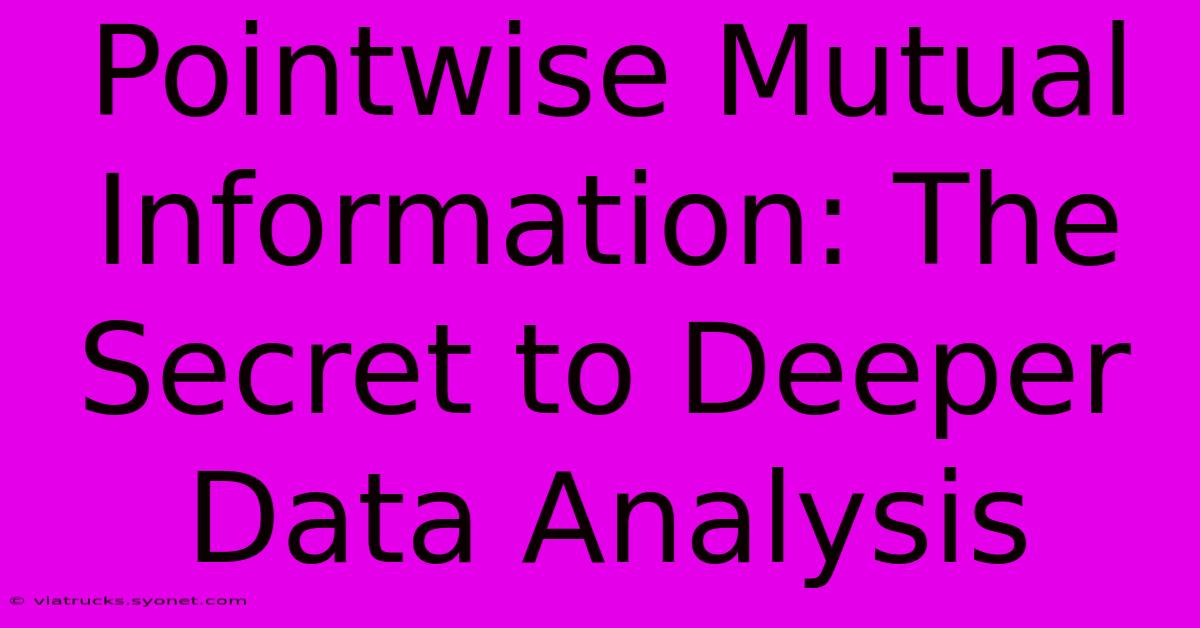
Table of Contents
Pointwise Mutual Information: The Secret to Deeper Data Analysis
Pointwise Mutual Information (PMI) might sound like a technical jargon only data scientists understand, but it's a powerful tool with the potential to unlock deeper insights from your data. This metric offers a unique way to quantify the relationship between two discrete random variables, revealing associations that other methods might miss. Understanding and applying PMI can significantly enhance your data analysis capabilities, whether you're working with text, images, or any other discrete data.
What is Pointwise Mutual Information?
At its core, PMI measures the statistical dependence between two events. It quantifies how much knowing about one event changes your knowledge about the other. A high PMI value indicates a strong association; a low value suggests weak or no association; and a negative value implies an inverse relationship.
Formally, the pointwise mutual information between two events, x and y, is defined as:
PMI(x, y) = log₂[P(x, y) / (P(x)P(y))]
Where:
- P(x, y) is the joint probability of events x and y occurring together.
- P(x) is the probability of event x occurring.
- P(y) is the probability of event y occurring.
The logarithm (base 2) is used to scale the result, making interpretation easier. A PMI of 0 indicates independence, while higher values represent stronger associations.
Understanding the Formula:
The formula essentially compares the observed joint probability P(x, y) with what you'd expect if x and y were independent, which is given by the product of their individual probabilities, P(x)P(y). If the observed joint probability is significantly higher than the expected probability, the PMI will be positive and large, indicating a strong association. Conversely, if the observed joint probability is lower, the PMI will be negative, indicating an inverse relationship or lack of association.
Applications of Pointwise Mutual Information
PMI's versatility makes it applicable across various domains:
1. Natural Language Processing (NLP):
PMI is extensively used in NLP for tasks like:
- Word association and collocation extraction: Identifying words that frequently appear together, revealing meaningful phrases and relationships within text corpora.
- Topic modeling: Discovering latent topics in a collection of documents by analyzing the co-occurrence of words.
- Sentiment analysis: Assessing the sentiment expressed in a text by examining the PMI between words and sentiment labels.
2. Image Analysis:
In image analysis, PMI can be used to:
- Feature extraction: Identifying co-occurring features within images, which can be valuable for image classification and object recognition.
- Image segmentation: Separating different regions in an image based on the statistical dependence of pixel values.
3. Bioinformatics:
PMI finds applications in bioinformatics for:
- Gene co-expression analysis: Identifying genes that are likely to be functionally related based on their expression patterns.
- Protein-protein interaction prediction: Predicting interactions between proteins based on their co-occurrence in biological pathways.
Advantages of Using PMI
- Intuitive Interpretation: The results are easy to understand and interpret, unlike some complex statistical measures.
- Versatility: It can be applied to a wide range of data types and analysis tasks.
- Efficiency: Computationally efficient for relatively small datasets.
Limitations of PMI
- Data Sparsity: PMI can be affected by data sparsity – if the joint probability of two events is very low or zero, the PMI calculation becomes unstable or undefined. Smoothing techniques are often necessary to mitigate this issue.
- Context Dependence: The meaning of PMI can be context-dependent. A high PMI between two events in one context may not necessarily imply a similar relationship in another context.
- Not a Distance Metric: Unlike some other similarity measures, PMI does not satisfy the properties of a distance metric.
Beyond the Basics: Addressing Data Sparsity
One of the most significant challenges when working with PMI is handling data sparsity. Rare co-occurrences can lead to inflated PMI values. Several techniques address this:
- Add-k smoothing: Adding a small constant (k) to all counts before calculating probabilities.
- Good-Turing smoothing: Estimating probabilities based on the frequency of unseen events.
- Kneser-Ney smoothing: A more sophisticated technique that accounts for the probability of unseen n-grams.
By employing appropriate smoothing techniques, you can obtain more robust and reliable PMI estimates, enhancing the accuracy of your data analysis.
Conclusion: Unlocking Insights with PMI
Pointwise Mutual Information provides a powerful lens for exploring relationships within your data. By carefully considering its strengths and limitations, and using appropriate smoothing techniques, you can leverage PMI to uncover hidden connections and gain a deeper understanding of the phenomena your data represents. Its application spans numerous fields, making it a valuable tool in any data analyst's arsenal. Remember to consider your specific dataset and research question when applying PMI to maximize its effectiveness.
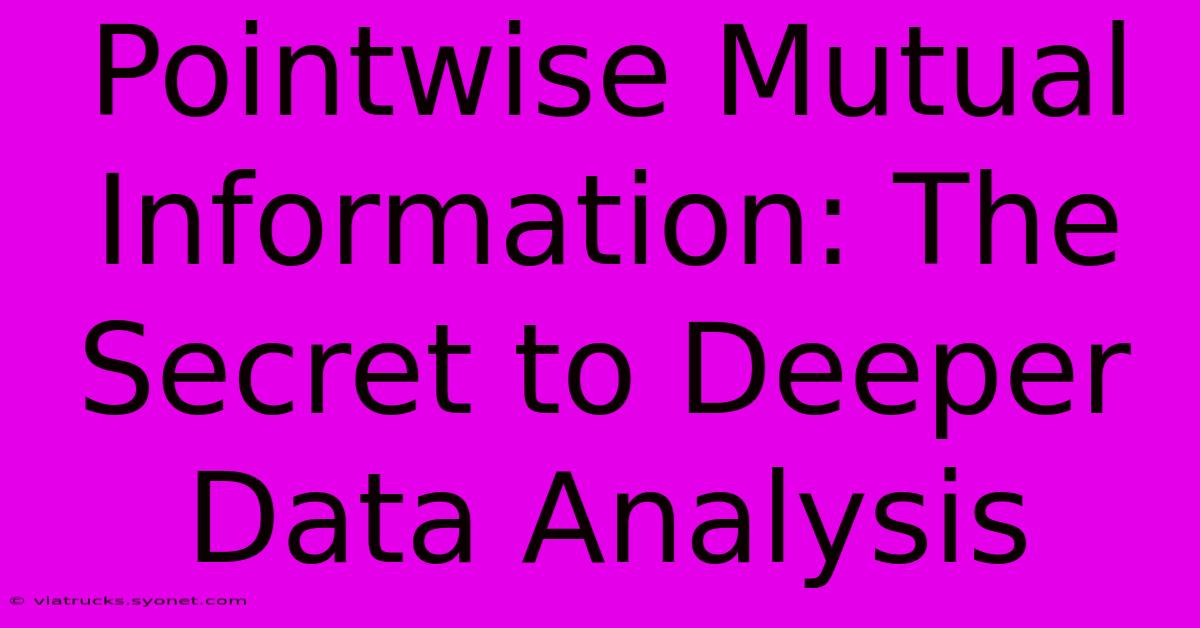
Thank you for visiting our website wich cover about Pointwise Mutual Information: The Secret To Deeper Data Analysis. We hope the information provided has been useful to you. Feel free to contact us if you have any questions or need further assistance. See you next time and dont miss to bookmark.
Featured Posts
-
Nba Game Preview 2 10 25 Warriors Bucks
Feb 09, 2025
-
Where Is Area Code 504 Find Out Now
Feb 09, 2025
-
Romanos Eye Injury Revealed
Feb 09, 2025
-
Overcome Procrastination A Day With Wilbur Robinson
Feb 09, 2025
-
Is Rick Moranis A Secret Millionaire Find Out Now
Feb 09, 2025