GMM: Unbiased Insights, Even With Imperfect Data
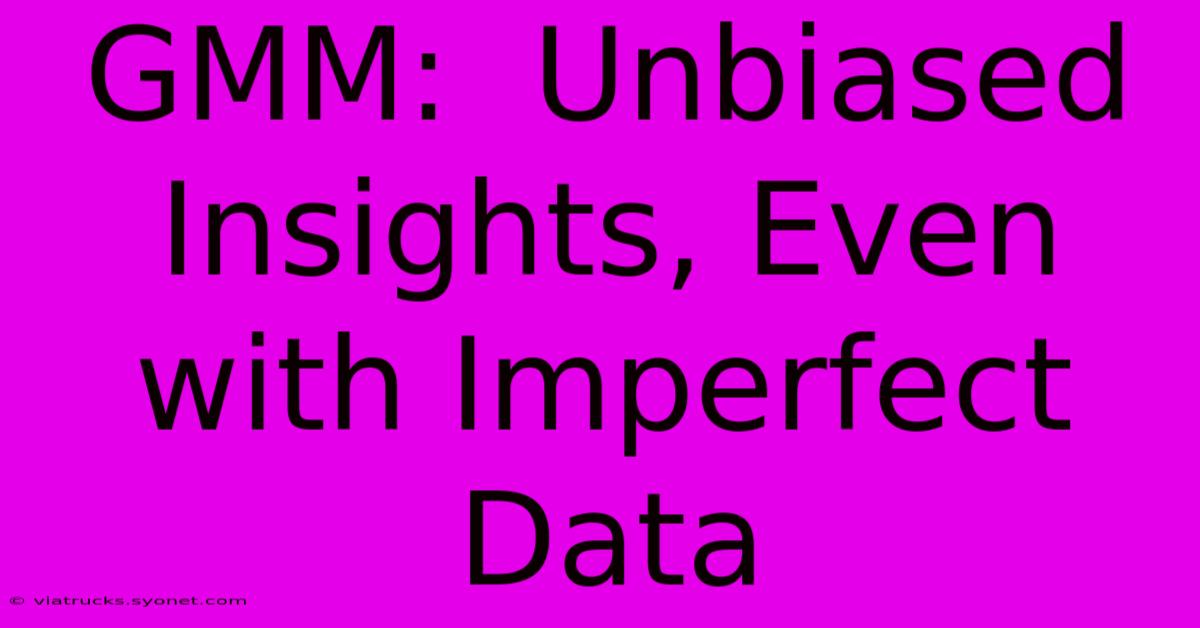
Table of Contents
GMM: Unbiased Insights, Even with Imperfect Data
Gaussian Mixture Models (GMMs) are powerful statistical tools used for clustering and density estimation. Unlike some other methods, GMMs offer a robust way to extract meaningful insights even when dealing with noisy or incomplete datasets. This article delves into the strengths of GMMs, exploring why they're a preferred choice for data analysis in various fields, despite the imperfections often inherent in real-world data.
Understanding Gaussian Mixture Models
At its core, a GMM assumes that the data points are generated from a mixture of several Gaussian distributions. Each Gaussian distribution represents a cluster, characterized by its own mean and covariance matrix. The model aims to identify the optimal number of clusters and their parameters, effectively separating the data into distinct groups. This process utilizes an iterative algorithm, often Expectation-Maximization (EM), to refine the cluster parameters until convergence.
Advantages of GMMs:
-
Handling Complex Data Distributions: Unlike simpler clustering algorithms like k-means, GMMs can model data with non-spherical clusters and varying densities. This flexibility is crucial for datasets that don't neatly fit into easily separable groups.
-
Probability-Based Clustering: GMMs assign probabilities to each data point belonging to each cluster. This soft clustering approach offers a more nuanced understanding compared to hard clustering methods that assign points to only one cluster. This probabilistic nature is particularly beneficial when dealing with overlapping clusters or uncertainty in data points.
-
Robustness to Noise and Outliers: While outliers can influence the results, the probabilistic nature of GMMs helps mitigate their impact significantly. The model doesn't solely rely on a single data point's location but incorporates the overall distribution to determine cluster boundaries.
-
Density Estimation: Besides clustering, GMMs excel at estimating the probability density function of the data. This provides a valuable tool for understanding the data's underlying structure and identifying potential anomalies.
GMMs and Imperfect Data: Why They Excel
Real-world datasets are rarely perfect. They often contain missing values, noise, and outliers. GMMs handle these imperfections surprisingly well, making them a reliable choice for a variety of applications:
Dealing with Missing Data:
GMMs can be adapted to handle missing data through techniques like Expectation-Maximization (EM) algorithms that iteratively estimate missing values while refining cluster parameters. This allows the model to effectively utilize all available information, maximizing the insights gleaned from incomplete datasets.
Managing Noise and Outliers:
The probabilistic nature of GMMs helps to dampen the influence of noisy data points and outliers. The model considers the overall data distribution when assigning points to clusters, minimizing the impact of individual anomalies.
Applications Across Diverse Fields:
The versatility and robustness of GMMs make them applicable in numerous fields:
-
Image Segmentation: GMMs are frequently used to segment images by clustering pixels based on color or texture features.
-
Financial Modeling: They can help identify different customer segments or predict market trends based on financial data.
-
Bioinformatics: GMMs are valuable in gene expression analysis and identifying distinct cell populations.
-
Speech Recognition: GMMs play a crucial role in modeling speech patterns and classifying different sounds.
Conclusion: Unlocking Insights with GMMs
Gaussian Mixture Models offer a powerful and versatile approach to data analysis, providing unbiased insights even when dealing with imperfect data. Their ability to handle complex data distributions, incorporate probabilistic information, and mitigate the effects of noise and outliers makes them a valuable tool for researchers and practitioners across numerous fields. By embracing the flexibility and robustness of GMMs, we can unlock valuable insights from even the most challenging datasets.
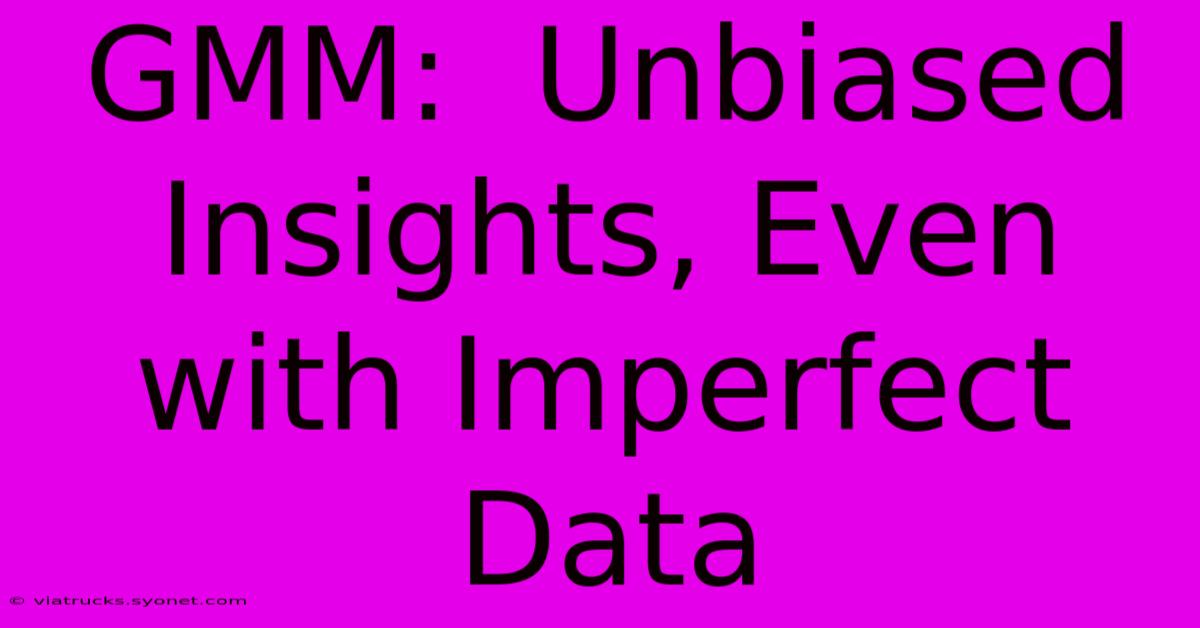
Thank you for visiting our website wich cover about GMM: Unbiased Insights, Even With Imperfect Data. We hope the information provided has been useful to you. Feel free to contact us if you have any questions or need further assistance. See you next time and dont miss to bookmark.
Featured Posts
-
Colorado Edges Oilers Necas Scores
Feb 09, 2025
-
Mark Williams Trade Rescinded
Feb 09, 2025
-
Bad Boys 4 Showtimes Skip The Lines Grab Your Seats
Feb 09, 2025
-
Experience The Magic Of The Firth Of Forth Estuary
Feb 09, 2025
-
Fa Cup Brighton Teen Chelsea Regstreeks
Feb 09, 2025