From Correlation To Causation? The Power Of PMI
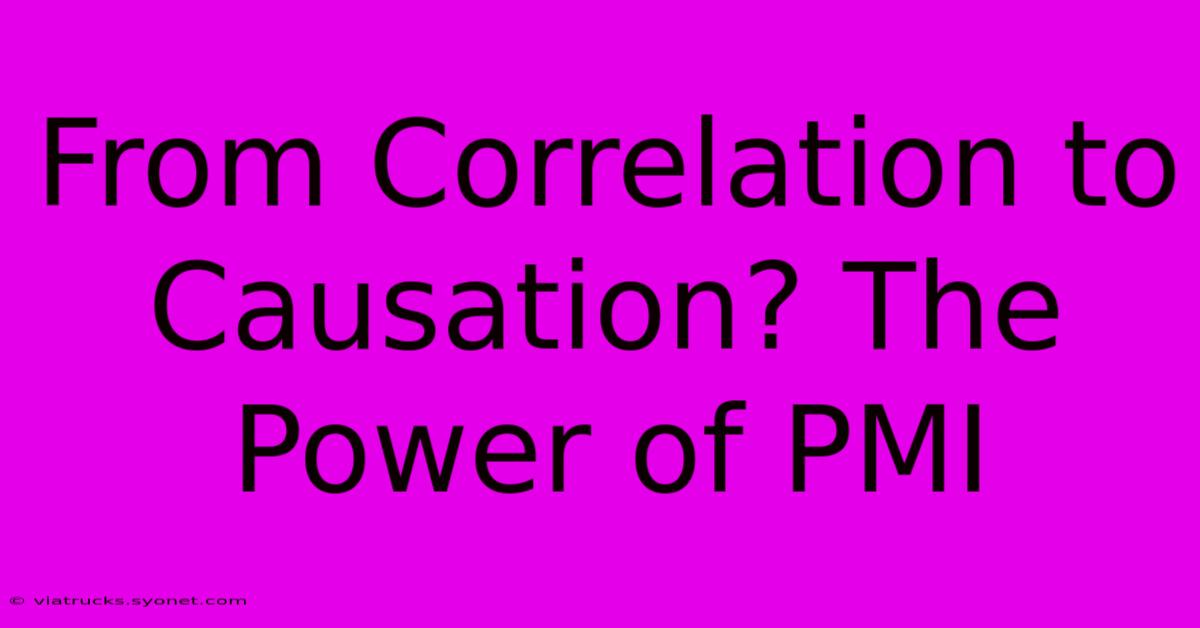
Table of Contents
From Correlation to Causation? The Power of PMI
Understanding the relationship between variables is crucial in many fields, from business analytics to scientific research. Often, we start by observing correlations – two variables that seem to move together. However, correlation doesn't equal causation. Just because two things happen together doesn't mean one causes the other. This is where PMI, or Pointwise Mutual Information, comes in. It helps us move beyond simple correlations and explore the potential for causal relationships.
What is Pointwise Mutual Information (PMI)?
PMI is a powerful statistical measure that quantifies the mutual dependence between two random variables. In simpler terms, it tells us how much knowing the value of one variable helps us predict the value of another. A high PMI suggests a strong relationship, while a low PMI indicates a weak or nonexistent relationship. Unlike correlation, which measures linear relationships, PMI can detect both linear and non-linear associations.
Understanding the Calculation
The PMI between two events, X and Y, is calculated using the following formula:
PMI(X;Y) = log₂[P(X,Y) / (P(X) * P(Y))]
Where:
- P(X,Y) is the joint probability of X and Y occurring together.
- P(X) is the probability of X occurring.
- P(Y) is the probability of Y occurring.
A positive PMI indicates that X and Y are more likely to occur together than would be expected by chance. A negative PMI suggests they are less likely to co-occur than expected by chance. A PMI of zero suggests independence – knowing the occurrence of one tells us nothing about the other.
PMI vs. Correlation: Key Differences
While both PMI and correlation coefficients (like Pearson's r) assess relationships between variables, they differ significantly:
Feature | PMI | Correlation (e.g., Pearson's r) |
---|---|---|
Relationship Type | Linear and non-linear | Primarily linear |
Scale | Unbounded (can be positive or negative) | Bounded between -1 and +1 |
Interpretation | Measures mutual information | Measures linear association strength |
Data Type | Categorical or continuous | Primarily continuous |
The Power of PMI: Applications and Examples
PMI finds applications across diverse fields:
1. Natural Language Processing (NLP):
PMI is extensively used in NLP to identify word associations and build semantic relationships. Analyzing the PMI between words helps in tasks like:
- Word sense disambiguation: Determining the correct meaning of a word based on its context.
- Collocation extraction: Identifying words that frequently appear together.
- Topic modeling: Discovering underlying themes in a large corpus of text.
2. Bioinformatics:
In bioinformatics, PMI helps in analyzing gene expression data, identifying gene co-regulation networks, and predicting protein-protein interactions.
3. Recommendation Systems:
PMI can be used to measure the association between items, leading to more accurate and personalized recommendations. For example, a high PMI between two movies suggests users who like one are likely to like the other.
4. Market Basket Analysis:
In retail, PMI assists in understanding customer purchasing behavior. By analyzing the PMI between different products, businesses can optimize product placement, develop targeted promotions, and improve sales strategies.
Limitations of PMI
While powerful, PMI has limitations:
- Sparsity: In datasets with many rare events, accurate estimation of probabilities can be challenging, leading to unreliable PMI values.
- Data Bias: PMI is sensitive to biases present in the data. If the dataset doesn't accurately represent the true underlying distribution, the PMI results may be misleading.
- Causation vs. Correlation: High PMI suggests a strong association, but it doesn't prove causation. Further investigation may be needed to establish causal relationships.
Conclusion: Unlocking Insights with PMI
PMI offers a valuable tool for analyzing relationships between variables, going beyond simple correlations. Its ability to capture both linear and non-linear associations makes it a powerful technique across various domains. While not a direct measure of causation, PMI can guide further investigations into potential causal links and unlock valuable insights from data. By carefully considering its limitations and applying it appropriately, PMI can be a powerful asset in your analytical toolkit.
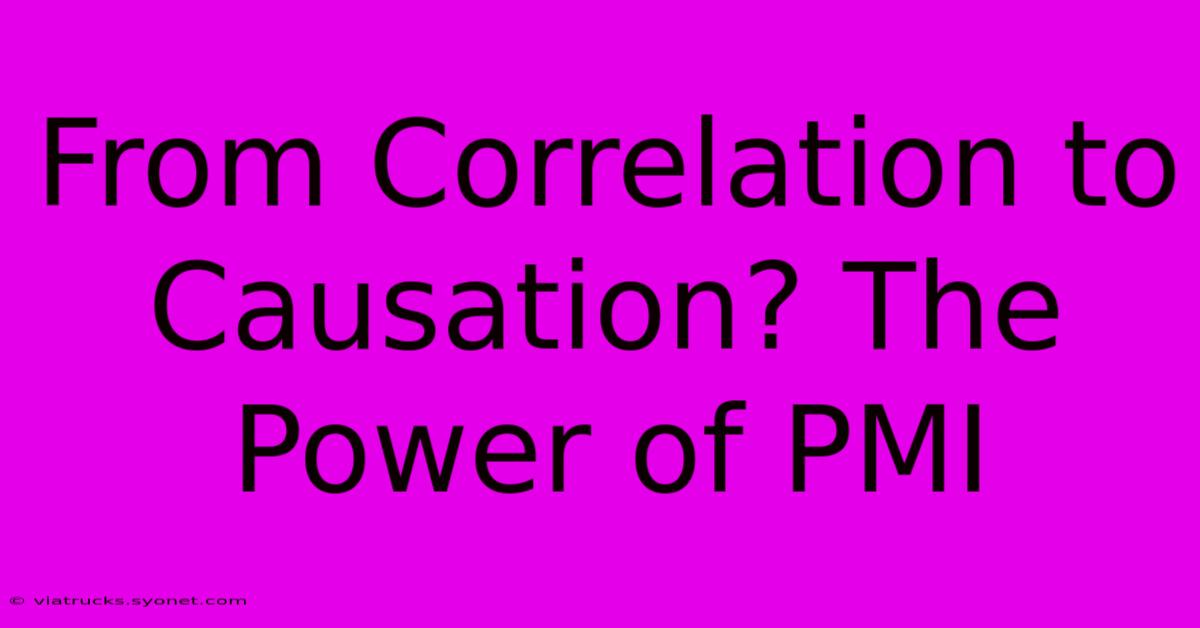
Thank you for visiting our website wich cover about From Correlation To Causation? The Power Of PMI. We hope the information provided has been useful to you. Feel free to contact us if you have any questions or need further assistance. See you next time and dont miss to bookmark.
Featured Posts
-
Lower Body Injury Ends Davis Mavericks Debut
Feb 09, 2025
-
From Coward To Courageous Learn From Coraje
Feb 09, 2025
-
Quien Cantara En El Super Bowl 2025
Feb 09, 2025
-
Chomo A Parents Guide To Online Safety
Feb 09, 2025
-
Finding Your Dream Home In Atwater Village
Feb 09, 2025