Conquering Complex Data With The Generalized Method Of Moments
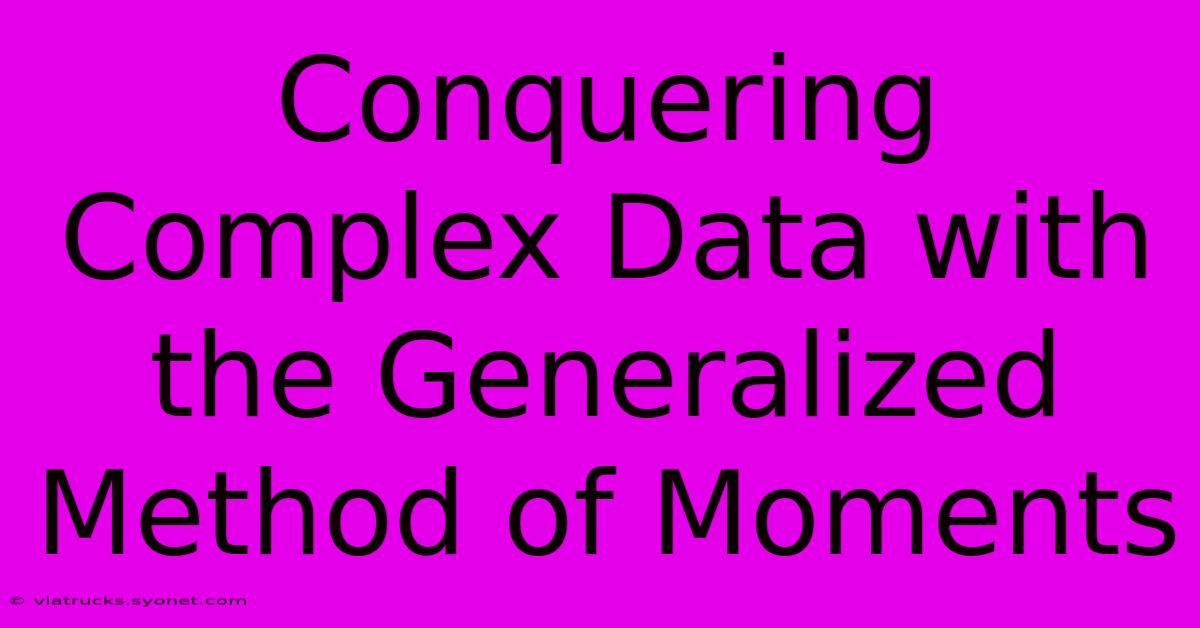
Table of Contents
Conquering Complex Data with the Generalized Method of Moments (GMM)
The world is awash in data. But raw data, without proper analysis, is just noise. Extracting meaningful insights from complex datasets often requires sophisticated statistical techniques, and among the most powerful is the Generalized Method of Moments (GMM). This article delves into GMM, explaining its applications, advantages, and limitations. We'll explore how it helps researchers overcome challenges posed by intricate data structures and unlock valuable information.
What is the Generalized Method of Moments?
GMM is a powerful econometric technique used to estimate parameters in statistical models. Unlike maximum likelihood estimation (MLE), GMM doesn't require specifying the full likelihood function. Instead, it leverages moment conditions, which are statements about the expected values of certain functions of the data and parameters. These moment conditions are derived from economic theory or prior knowledge about the data-generating process.
Key Advantages of GMM:
-
Flexibility: GMM is incredibly versatile, handling various types of data, including time series, panel data, and cross-sectional data. Its ability to work with non-normal data makes it a preferred choice when dealing with complex datasets exhibiting skewness or heavy tails.
-
Robustness: GMM is robust to misspecification of the error distribution. Unlike methods that assume specific error distributions (like normality), GMM provides consistent estimates even if the assumptions about the error terms are violated.
-
Efficiency: While not always the most efficient estimator, GMM offers optimal efficiency under certain conditions. This is achieved through the use of a weighting matrix, which is carefully chosen to minimize the variance of the estimates.
How GMM Works:
GMM works by minimizing a distance metric between the sample moments and the theoretical moments implied by the model. This involves:
-
Formulating Moment Conditions: The first crucial step is defining the moment conditions based on the model and available information. These conditions represent restrictions on the expected values of certain functions of the data.
-
Estimating Sample Moments: The next step is calculating the sample analogs of the theoretical moments using the observed data.
-
Choosing a Weighting Matrix: The weighting matrix plays a critical role in improving the efficiency of the GMM estimator. Optimal weighting matrices can be obtained using various methods.
-
Minimizing the Distance: Finally, GMM finds the parameter values that minimize the weighted distance between the sample moments and the theoretical moments. This minimization is typically achieved using numerical optimization techniques.
Applications of GMM:
GMM finds widespread application in diverse fields:
-
Econometrics: Estimating parameters in dynamic panel data models, instrumental variable regression, and models with endogenous variables.
-
Finance: Modeling asset pricing, analyzing portfolio returns, and estimating risk factors.
-
Biostatistics: Analyzing longitudinal data, modeling survival data, and estimating treatment effects.
-
Other Disciplines: GMM has applications in areas like environmental science, epidemiology, and engineering.
Limitations of GMM:
Despite its power, GMM has limitations:
-
Weak Instruments: When the instruments are weakly correlated with the endogenous variables, the GMM estimates can be biased and have large standard errors.
-
Computational Intensity: Implementing GMM often involves numerical optimization, which can be computationally intensive, especially with large datasets and complex models.
-
Sensitivity to Weighting Matrix: The choice of weighting matrix significantly impacts the efficiency of the GMM estimator. An inappropriate choice can lead to less efficient estimates.
Conclusion:
The Generalized Method of Moments is a versatile and robust technique for analyzing complex data. Its ability to handle various data types and its robustness to misspecification makes it a valuable tool in many fields. While challenges exist, particularly with weak instruments, the power and flexibility of GMM make it an indispensable statistical method for researchers seeking to extract valuable insights from intricate datasets. By carefully formulating moment conditions and selecting an appropriate weighting matrix, researchers can leverage GMM's capabilities to conquer complex data and unlock new knowledge.
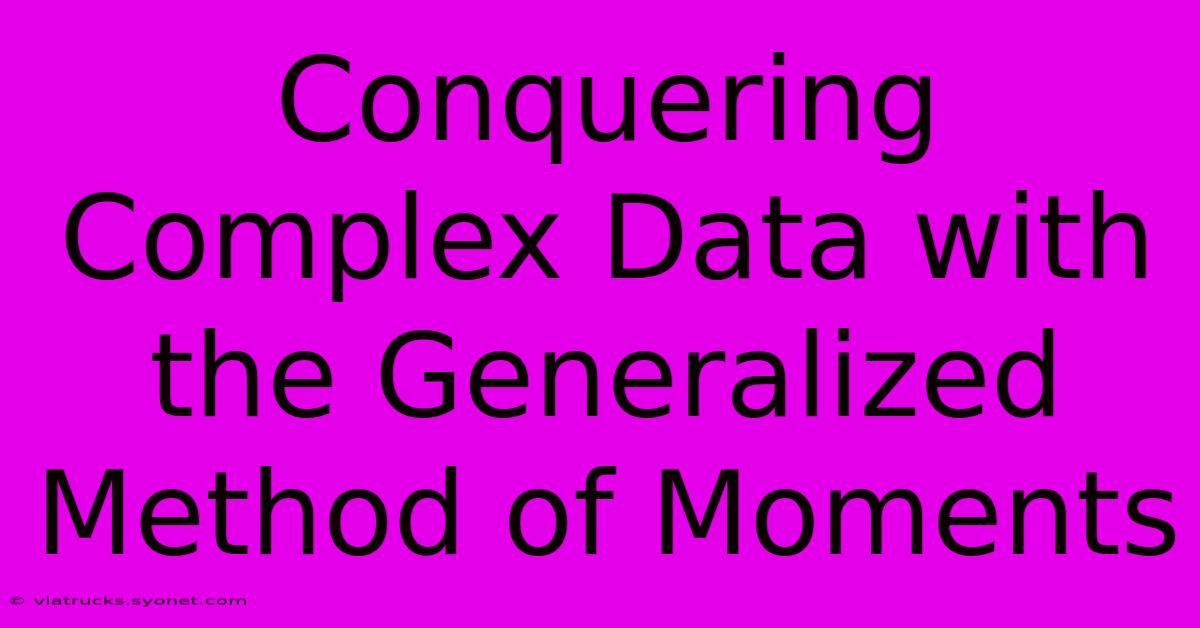
Thank you for visiting our website wich cover about Conquering Complex Data With The Generalized Method Of Moments. We hope the information provided has been useful to you. Feel free to contact us if you have any questions or need further assistance. See you next time and dont miss to bookmark.
Featured Posts
-
Real Madrid 1 1 Atletico La Liga Match
Feb 09, 2025
-
Mick Jones The Clashs Unsung Hero Finally Speaks
Feb 09, 2025
-
Quick Cash The Ultimate Sidewalk Dice Game
Feb 09, 2025
-
Espn Analysis Miami 5 0 Olimpia
Feb 09, 2025
-
Fa Cup Var Absence In Fourth Round
Feb 09, 2025