Beyond Simple Averages: The Power Of GMM
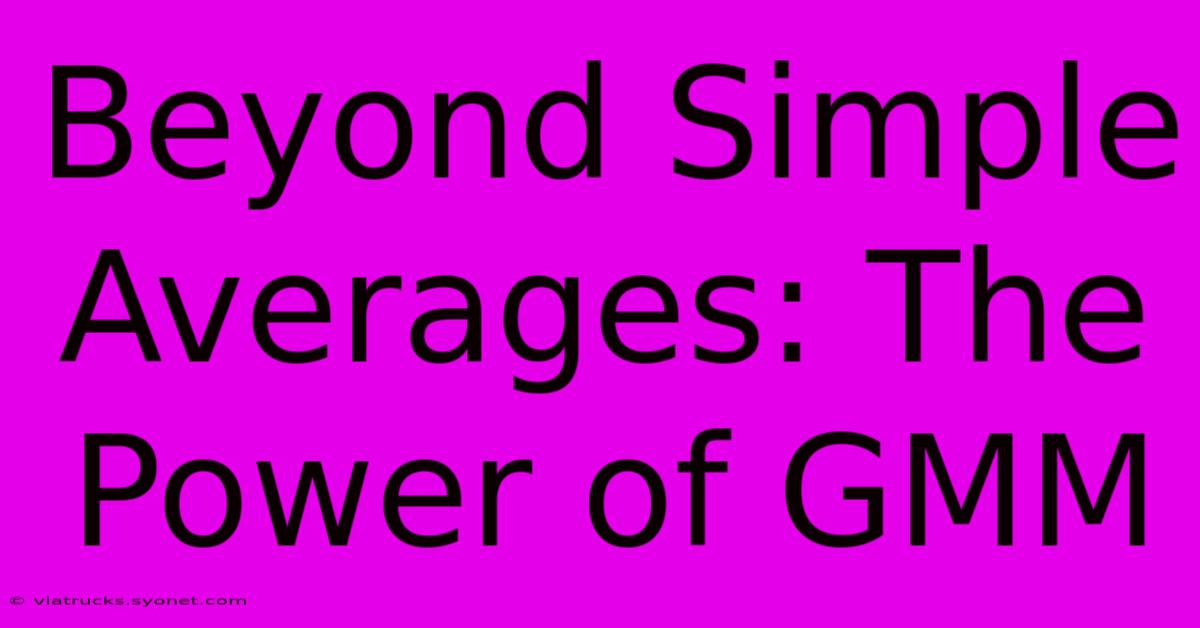
Table of Contents
Beyond Simple Averages: The Power of Gaussian Mixture Models (GMM)
For many data analysis tasks, a simple average provides a sufficient summary. However, when dealing with data exhibiting underlying structure or multiple subpopulations, the limitations of a single average become apparent. This is where Gaussian Mixture Models (GMMs) shine. GMMs offer a powerful and flexible approach to model data that isn't easily captured by simpler methods. This article delves into the capabilities of GMMs, exploring their applications and advantages over traditional averaging techniques.
Understanding the Limitations of Simple Averages
A simple average, while easy to calculate and understand, masks crucial information when your data comprises distinct groups. Imagine calculating the average income of a population that includes both high-income earners and low-income earners. The resulting average will obscure the bimodal nature of the income distribution, failing to represent the reality of two distinct subgroups within the population. This is precisely where GMMs offer a superior solution.
What are Gaussian Mixture Models (GMMs)?
GMMs are probabilistic models that assume the data is generated from a mixture of multiple Gaussian distributions. Each Gaussian distribution represents a distinct subpopulation or cluster within the data. The model learns the parameters of each Gaussian (mean, variance, and weight) to best fit the observed data. This allows for a more nuanced understanding of the underlying data structure than simple averages can provide.
Key Components of a GMM:
- Gaussian Distributions: Each component of the mixture is a Gaussian (normal) distribution, characterized by its mean and covariance matrix.
- Mixing Weights: These weights represent the proportion of data points belonging to each Gaussian component. They sum to one.
- Expectation-Maximization (EM) Algorithm: This iterative algorithm is typically used to estimate the parameters of the GMM. It alternates between estimating the cluster assignments (expectation step) and updating the parameters of each Gaussian (maximization step).
Advantages of Using GMMs
GMMs offer several significant advantages over simple averaging techniques:
- Uncovering Hidden Structure: GMMs excel at identifying clusters or subpopulations within data, revealing patterns invisible to simple averages.
- Improved Accuracy in Modeling: By accounting for the multiple underlying distributions, GMMs provide a more accurate representation of the data's complexity, leading to more accurate predictions and classifications.
- Handling Multimodal Data: Unlike simple averages, GMMs effectively handle data with multiple modes (peaks) in its distribution.
- Flexibility and Adaptability: GMMs can be applied to a wide range of data types and dimensions, making them a versatile tool for data analysis.
Applications of GMMs
GMMs find applications across diverse fields:
- Clustering: Identifying groups of similar data points in unsupervised learning scenarios. Examples include customer segmentation, image segmentation, and anomaly detection.
- Density Estimation: Estimating the probability density function of the data, providing insights into data distribution.
- Classification: Assigning data points to predefined classes based on their probability of belonging to each Gaussian component.
- Signal Processing: Analyzing and separating overlapping signals, such as in speech recognition and biomedical signal processing.
Beyond the Basics: Advanced Techniques and Considerations
While the basic GMM framework is powerful, several advanced techniques can further enhance its capabilities. These include:
- Model Selection: Determining the optimal number of Gaussian components is crucial. Techniques like the Bayesian Information Criterion (BIC) or Akaike Information Criterion (AIC) can assist in this process.
- Handling High-Dimensional Data: Dimensionality reduction techniques can be employed to address the computational challenges associated with high-dimensional data.
- Dealing with Outliers: Robust estimation techniques can mitigate the influence of outliers on the GMM parameters.
Conclusion: Embracing the Power of GMMs
Simple averages, while convenient, often fail to capture the richness and complexity inherent in many datasets. Gaussian Mixture Models offer a sophisticated alternative, providing a more nuanced and accurate understanding of data structure. Their ability to uncover hidden patterns, handle multimodal distributions, and provide a probabilistic framework makes them an invaluable tool in various data analysis applications. By moving beyond simple averages and embracing the power of GMMs, researchers and analysts can unlock deeper insights from their data and make more informed decisions.
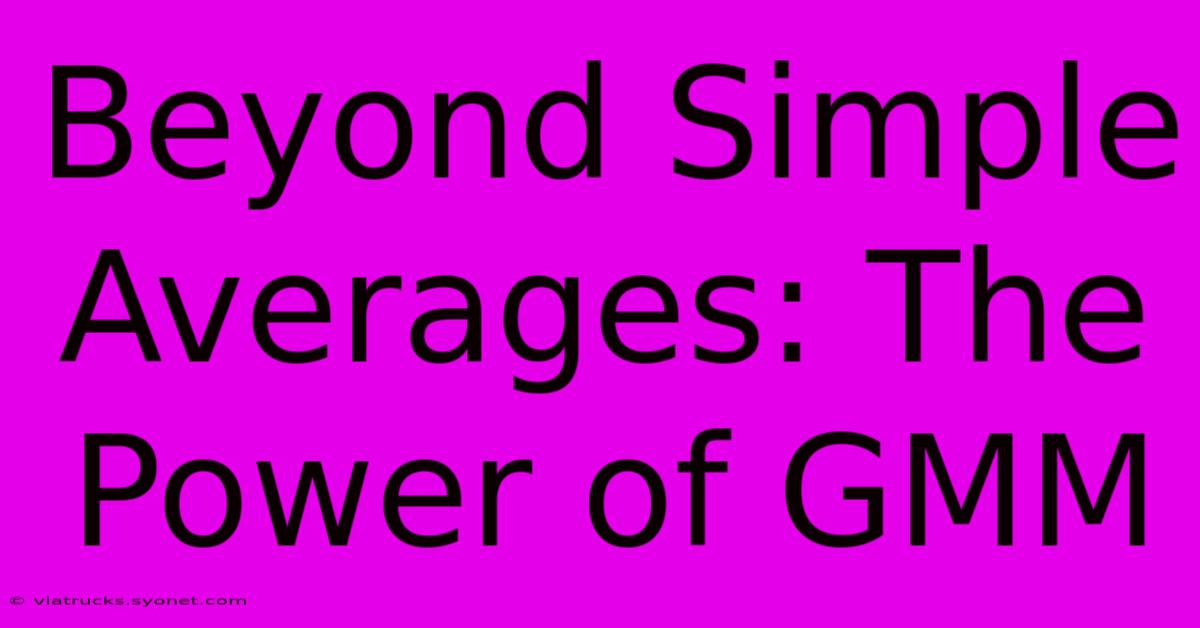
Thank you for visiting our website wich cover about Beyond Simple Averages: The Power Of GMM. We hope the information provided has been useful to you. Feel free to contact us if you have any questions or need further assistance. See you next time and dont miss to bookmark.
Featured Posts
-
Green Bay Packers Standings Update And Playoff Picture
Feb 09, 2025
-
Keasbey Nj Community Culture And Connection In The United States
Feb 09, 2025
-
Mavericks Vs Rockets 2025 Nba Odds
Feb 09, 2025
-
Rahnmay Kaml Jng Ayran W Asrayyl
Feb 09, 2025
-
Conquer Your Demons 44 Days To A New You
Feb 09, 2025