Beyond Correlation: Understanding Pointwise Mutual Information
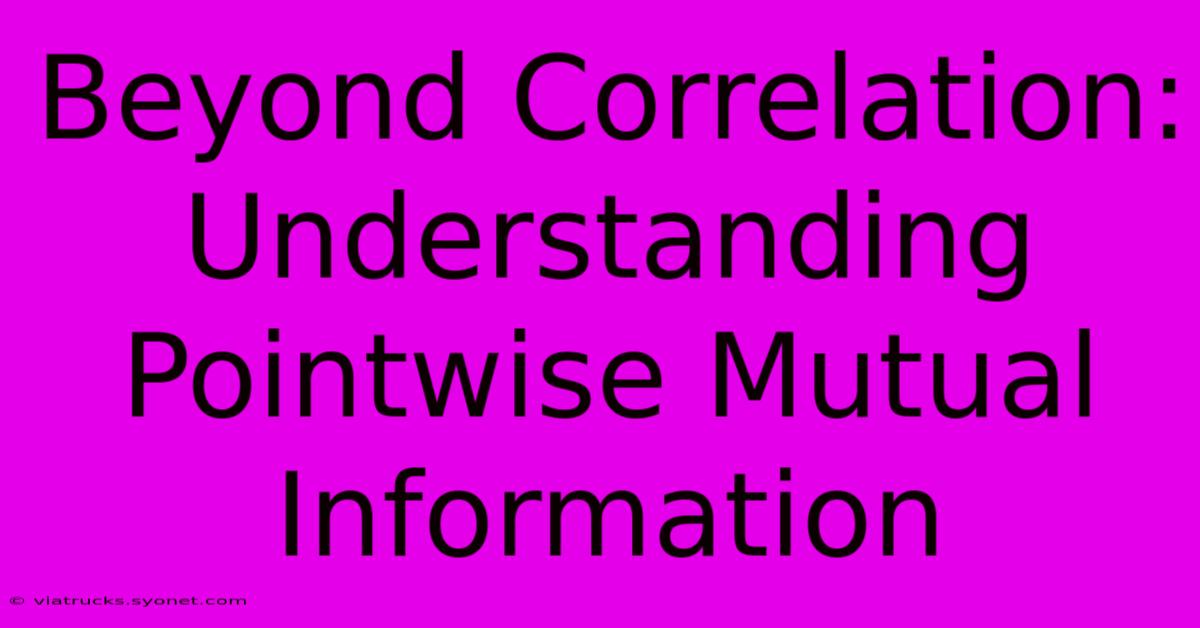
Table of Contents
Beyond Correlation: Understanding Pointwise Mutual Information
Correlation is a familiar concept in statistics, providing a measure of the linear relationship between two variables. However, correlation alone often falls short in capturing the complex dependencies that exist within data, especially when dealing with non-linear relationships. This is where Pointwise Mutual Information (PMI) steps in, offering a more nuanced understanding of the relationship between individual data points. This article delves into the intricacies of PMI, exploring its applications and limitations.
What is Pointwise Mutual Information (PMI)?
PMI quantifies the association between two events, x
and y
. Unlike correlation, which focuses on the overall linear relationship, PMI measures the pointwise association – the relationship between specific instances of x
and y
. It's based on the concept of mutual information, which measures the reduction in uncertainty about one variable given knowledge of another.
In simpler terms, PMI tells us how much knowing one data point changes our understanding of the probability of seeing another specific data point. A high PMI indicates a strong association, meaning the occurrence of one event significantly increases the likelihood of the other. Conversely, a low PMI suggests a weak or even negative association.
The formula for PMI is:
PMI(x, y) = log₂[P(x, y) / (P(x) * P(y))]
Where:
P(x, y)
is the joint probability of events x and y occurring together.P(x)
is the probability of event x occurring.P(y)
is the probability of event y occurring.
The logarithm (base 2) is used to express the information in bits. A positive PMI indicates that the events are more likely to occur together than expected by chance, while a negative PMI indicates the opposite. A PMI of 0 suggests the events are independent.
Interpreting PMI Values
- PMI > 0: The co-occurrence of x and y is more frequent than expected by chance. They are positively associated.
- PMI = 0: The co-occurrence of x and y is exactly what would be expected by chance. They are independent.
- PMI < 0: The co-occurrence of x and y is less frequent than expected by chance. They are negatively associated.
PMI vs. Correlation: Key Differences
While both PMI and correlation assess relationships between variables, they do so in fundamentally different ways:
Feature | Pointwise Mutual Information (PMI) | Correlation |
---|---|---|
Nature | Measures pointwise association | Measures linear association |
Relationship | Captures non-linear relationships | Primarily captures linear relationships |
Data Type | Categorical or numerical | Primarily numerical |
Scale | Unbounded | Bounded between -1 and 1 |
Applications of Pointwise Mutual Information
PMI finds applications in various fields:
- Natural Language Processing (NLP): Determining word associations, identifying collocations (words frequently appearing together), and improving word embedding models. For example, PMI helps understand the strength of the association between words like "artificial" and "intelligence."
- Information Retrieval: Ranking documents based on the relevance of keywords. Higher PMI between a query term and a document's terms signifies greater relevance.
- Bioinformatics: Analyzing gene expression data, identifying co-occurring genes, and understanding biological pathways.
- Recommendation Systems: Identifying item pairs frequently purchased together to suggest products to users.
Limitations of Pointwise Mutual Information
Despite its advantages, PMI has certain limitations:
- Sensitivity to low probabilities: PMI can be heavily influenced by low probability events, leading to unstable estimates. Smoothing techniques are often employed to mitigate this issue.
- Sparsity issues: In high-dimensional data, many joint probabilities may be zero, making PMI calculations unreliable.
- Does not capture directionality: PMI indicates association but doesn't reveal the direction of the relationship. For instance, a high PMI between "rain" and "umbrella" doesn't imply that rain causes people to use umbrellas.
Conclusion
Pointwise Mutual Information offers a powerful tool for analyzing the relationships between data points, going beyond the limitations of correlation. Its ability to capture non-linear dependencies makes it valuable in diverse fields. However, it is crucial to be aware of its limitations and apply appropriate techniques to ensure reliable results. Understanding both the strengths and weaknesses of PMI is key to its effective implementation in data analysis.
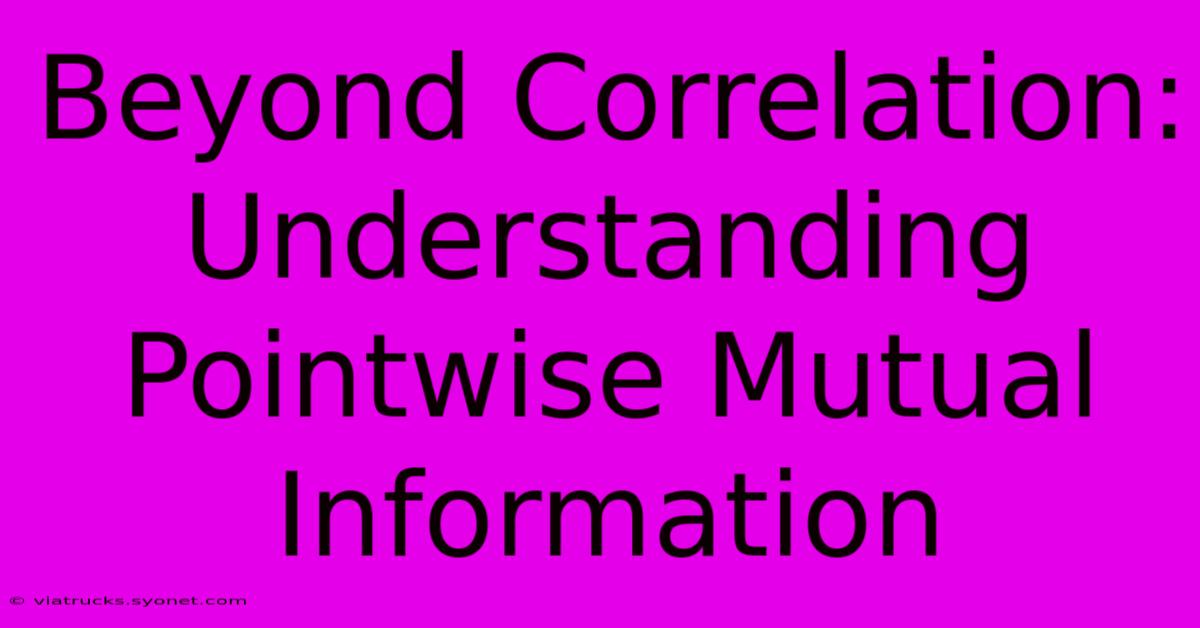
Thank you for visiting our website wich cover about Beyond Correlation: Understanding Pointwise Mutual Information. We hope the information provided has been useful to you. Feel free to contact us if you have any questions or need further assistance. See you next time and dont miss to bookmark.
Featured Posts
-
Beyond The 90 Minutes The Perks Of Being A Santa Fe Socio
Feb 09, 2025
-
Brighton Vs Chelsea Fourth Round Teams
Feb 09, 2025
-
Best Of Both Worlds Tour Two Destinations One Unforgettable Trip
Feb 09, 2025
-
Johnny And The Sprites Find Your Inner Sparkle
Feb 09, 2025
-
Puchner Silber Nach Magerem Winter
Feb 09, 2025